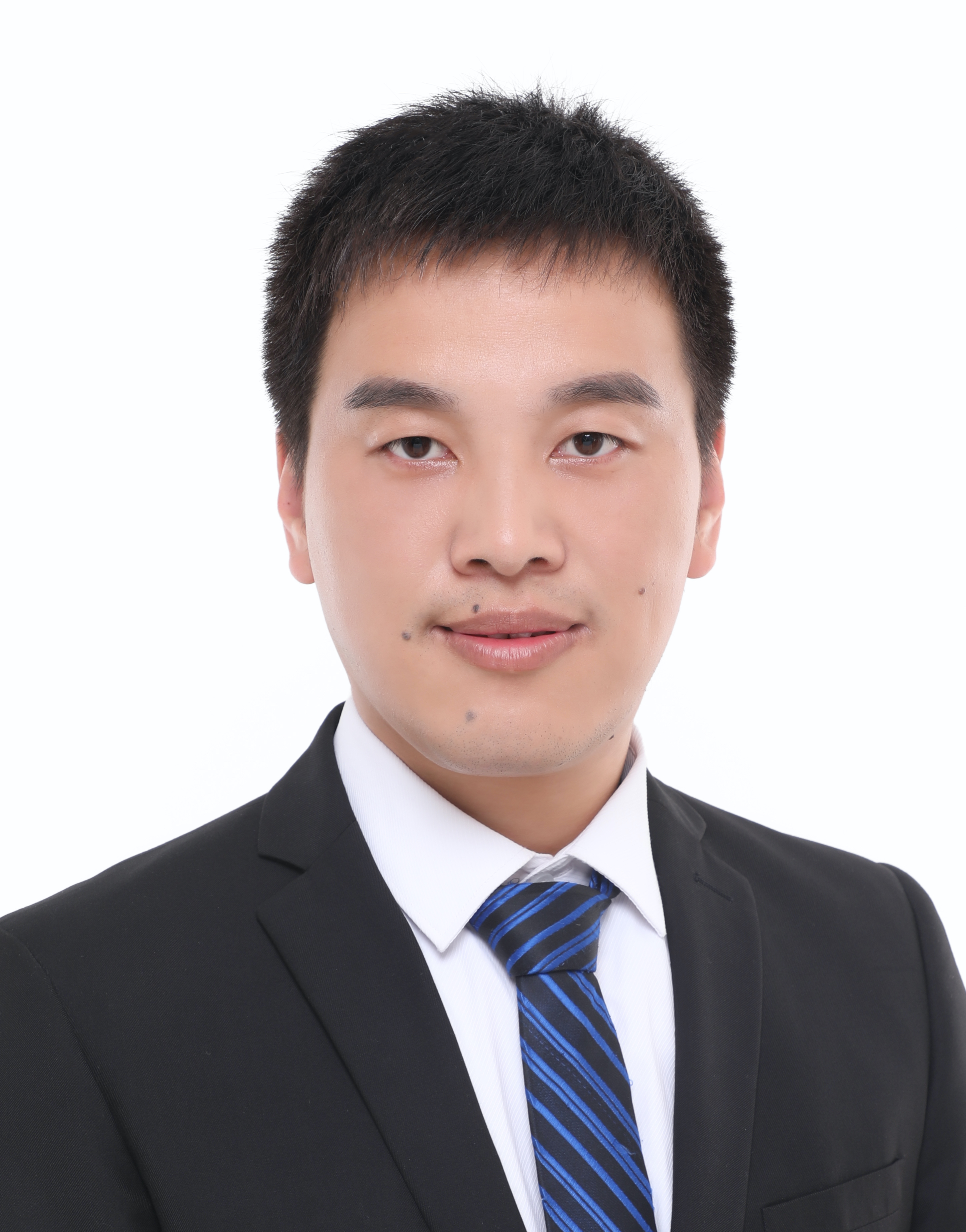
Wenshuo Wang
Postdoc, McGill University
Date: 11/21, 2022
Topic: Uncover Human-Driving Behavioral Interaction Patterns Toward Socially Autonomous Mobility
Bio: Wenshuo Wang received his Ph.D. degree in Mechanical Engineering, Beijing Institute of Technology (BIT), China in 2018. He was a Postdoc Associate at UC Berkeley and Carnegie Mellon University from 2018-2020 and a visiting graduate student at UC Berkeley and the University of Michigan from 2015-2018. He is now working as a Postdoc at McGill University. He received the George N. Saridis Best Transactions Paper Award (2022), Global Young Rising Star Scholar in ‘AI+X’ (2022), IVADO Postdoc Fellowship Award (2020), SAE-China Best Ph.D. Dissertation Award (2018), and Early Career Ph.D. Fellowship (2017). Now he serves as an Associate Editor for the Journal of Field Robotics, IEEE Transactions on Intelligent Vehicles, and IEEE Transactions on Vehicular Technology. Dr. Wang’s research goal is to develop theoretical frameworks and practical algorithms to understand human interactions by employing Bayesian learning and optimization with the support of computational cognitive sciences toward Socially Interactive Autonomous Mobility, a truly safe and trustworthy system that places the human at the center of a superior driving experience.
Abstract: No human drives a car in a vacuum; she/he must negotiate with other road users to achieve their goals in social traffic scenes. Nowadays, autonomous vehicles face significant challenges in making safe and efficient decisions when interacting with other human road users (human drivers, pedestrians, and cyclists). AVs should behave socially such that their behaviors and actions are safe and predictable even without explicit communication. In this talk, I will first define social interaction in road traffic specified in a computationally practical way by leveraging dynamics, measurement, and optimization. Then, I will introduce a primitive-based concept to semantically understand the dynamic behavioral interactions among multiple traffic agents using nonparametric Bayesian learning, which helps insight into the behavioral interaction patterns and design associated practical algorithms for socially autonomous vehicles. Finally, I will show some related applications of driving primitives in behavior similarity quantification, scenario generation, decision-making, and policy learning.
Bio: Wenshuo Wang received his Ph.D. degree in Mechanical Engineering, Beijing Institute of Technology (BIT), China in 2018. He was a Postdoc Associate at UC Berkeley and Carnegie Mellon University from 2018-2020 and a visiting graduate student at UC Berkeley and the University of Michigan from 2015-2018. He is now working as a Postdoc at McGill University. He received the George N. Saridis Best Transactions Paper Award (2022), Global Young Rising Star Scholar in ‘AI+X’ (2022), IVADO Postdoc Fellowship Award (2020), SAE-China Best Ph.D. Dissertation Award (2018), and Early Career Ph.D. Fellowship (2017). Now he serves as an Associate Editor for the Journal of Field Robotics, IEEE Transactions on Intelligent Vehicles, and IEEE Transactions on Vehicular Technology. Dr. Wang’s research goal is to develop theoretical frameworks and practical algorithms to understand human interactions by employing Bayesian learning and optimization with the support of computational cognitive sciences toward Socially Interactive Autonomous Mobility, a truly safe and trustworthy system that places the human at the center of a superior driving experience.
Abstract: No human drives a car in a vacuum; she/he must negotiate with other road users to achieve their goals in social traffic scenes. Nowadays, autonomous vehicles face significant challenges in making safe and efficient decisions when interacting with other human road users (human drivers, pedestrians, and cyclists). AVs should behave socially such that their behaviors and actions are safe and predictable even without explicit communication. In this talk, I will first define social interaction in road traffic specified in a computationally practical way by leveraging dynamics, measurement, and optimization. Then, I will introduce a primitive-based concept to semantically understand the dynamic behavioral interactions among multiple traffic agents using nonparametric Bayesian learning, which helps insight into the behavioral interaction patterns and design associated practical algorithms for socially autonomous vehicles. Finally, I will show some related applications of driving primitives in behavior similarity quantification, scenario generation, decision-making, and policy learning.